
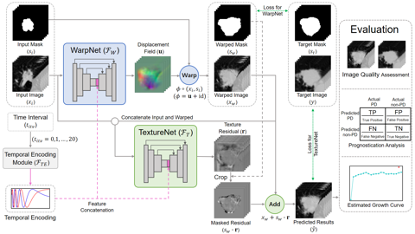
Malignancy-Aware Follow-Up Volume Prediction for Lung Nodules
- Date:2020/06/23
- Team: Yamin Li, Jiancheng Yang, Yi Xu, Jingwei Xu
- Goal: We aim at a unified framework to predict future volume of a lung nodule, given any time interval and a baseline volume.
Brief Introduction
To model the growth of nodules, we develop a Nodule Follow-Up Prediction Network (NoFoNet, see the figure.) consisting of a WarpNet and a TextureNet for spatial and texture (intensity) transformations respectively, where an integrated temporal encoding module (TEM) is addressed to encode different follow-up time interval information into the lesion representation.
To the best of our knowledge, this is the first study to predict nodule growth quantitatively (size) and visually (appearance) given any time interval during. The proposed NoFoNet could produce both high-quality visual appearances and accurate quantitative clinical assessment for lung nodule follow-up. Our in-house follow-up dataset from two medical centers validates the effectiveness of the framework.
Future works:
Most nodules in our current dataset have no pathological information, so it is difficult to accurately explore the differences between the prediction results of malignant and benign nodules. We will collect more nodules with pathology information to improve the experimental results. Meanwhile, we are exploring more efficient and effective architectures and temporal encoding methods of our framework, with more follow-up nodules collected latter.
Results:
Continuous prediction results: Vary the discretized time interval
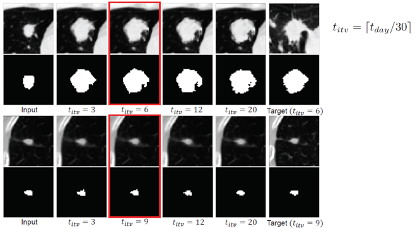
Comparative results: Between our NoFoNet and baseline U-Net
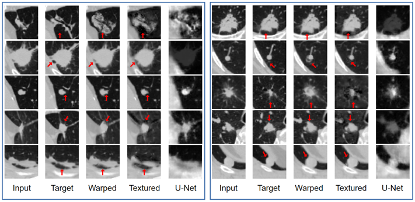
Paper
PDF: https://arxiv.org/pdf/2006.13890.pdf