
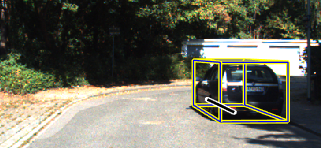
Intelligent Assisted Driving -- 3D Vehicles Detection
- Date:25/12/17
- Team: Yi Xu, Siyu wan
- Goal: To achieve intelligent assisted driving system, which can accurately detect and recognize the vehicles
Brief Introduction
3D detection is a crucial problem in autonomous driving scenarios which involves perception or interaction with other vehicles leveraging images or LIDAR point clouds.Fusion between image and point cloud should give better performance. We create a two-stream feature fusion network to predict 2D and 3D bounding box jointly from raw point cloud and corresponding images in real-time. All parts of our model could be train in a end-to-end manner. For better training, we further proposed a projection consistency loss to capture the structure between 2D and 3D prediction.
In the process of vehicles detection, the accurate detection and recognition of objects with different scales. The 2D vehicles detection has a good performance on multi-scales but has fewer informations. The 3D vehicles detection has more informations but the point cloud is very sparse and provide less information for the far objects. We conduct a feature fusion network to concat the 2D features and 3D features to achieve a better performance.
The vehicle detection algorithm extracts the vehicle target from the image sequence and locates it. It is a problem of object recognition and motion detection in computer vision. By combining 2D vehicle detection and 3D vehicle detection, we can more accurately detect the spatial position and posture of the vehicle by concat 2D and 3D features of the vehicle.
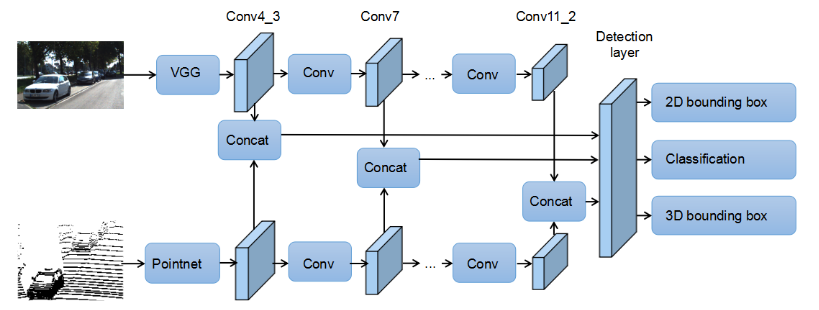
Paper
Coming soon!
Dataset
Coming soon!